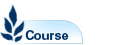
Health Data Analytics: Statistical Modelling II - HDAT9700
Faculty: Faculty of Medicine
School: School of Medical Sciences
Course Outline: MSc Health Data Science
Campus: Sydney
Career: Postgraduate
Units of Credit: 6
EFTSL: 0.12500 (more info)
Indicative Contact Hours per Week: 10
CSS Contribution Charge: 3 (more info)
Tuition Fee: See Tuition Fee Schedule
Further Information: See Class Timetable
View course information for previous years.
Description
This course builds upon Health Data Analytics: Statistical Modelling I (HDAT9600), expanding the Generalised Linear Model (GLM) to the more powerful and even more flexible Generalised Linear Mixed-effects Model (GLMM). GLMMs are often known as mixed-effects, random-effects, multilevel, or hierarchical models.
Simple hierarchies encountered in health data are first outlined, highlighting the assumptions and consequences of ignoring such hierarchy. Increasingly sophisticated model specifications are introduced. This sophistication is illustrated through the appropriate modelling of repeated measures (longitudinal) data, including time-varying covariates. A spectrum of model diagnostics and comparisons, both algorithmic and graphical, are used to consider the suitability of GLMM for health research. The role of contextual effects and interactions within the framework is explored. Alternative estimation (MCMC) techniques and outcome distributions appropriate for health data are considered. Cross-classified and multiple-membership models illustrate the flexibility to relax the strict hierarchy. Building from non-parametric, through semi-parametric (proportional hazards), to parametric (relative survival) models, a practical application of survival analysis will be posed as a GLMM. The advantages and limitations of GLMM for health research will be considered.
The course concludes with an overview of some common statistical pitfalls (statistical interaction and collinearity, regression to the mean, reversal paradox, mathematical coupling), with examples drawn from the health research literature.
The main processes used to drive the content will be a flipped classroom using short instructional videos covering the content, integrated with extensive face-to-face tutorials and workshops. Peer instruction will be utilised both during face to-face sessions and (private) group learning activities. Active and self-directed learning will be supported via the Moodle TELT. All statistical concepts will be illustrated with a variety of health examples.
2. Construct GLMMs with appropriate data structures representing natural/ biological hierarchies.
3. Appraise model fit using a variety of model diagnostics.
4. Compose narratives of GLMM interpretation within the framework of statistical inference.
5. Visualise statistical techniques as special parameterisations of the GLMM.
Tutorials: 2 hours
Web-based online learning activities: 7 hours