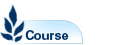
Health Data Analytics: Statistical Modelling I - HDAT9600
Faculty: Faculty of Medicine
School: School of Medical Sciences
Course Outline: MSc Health Data Science
Campus: Sydney
Career: Postgraduate
Units of Credit: 6
EFTSL: 0.12500 (more info)
Indicative Contact Hours per Week: 10
CSS Contribution Charge: 3 (more info)
Tuition Fee: See Tuition Fee Schedule
Further Information: See Class Timetable
View course information for previous years.
Description
This course provides a sound grounding in computation for Health Data Science, specifically in the flexible methods of generalised linear modelling (GLM). Building from (simple) linear regression, modelling building techniques are developed and posed within the more general framework of GLMs. The utility of automated selection procedures will be contrasted with Directed Acyclic Graphs (DAGs), for the identification of confounding sets in health research. Model diagnostics and comparisons (Fisher and observed information, likelihood ratio, Wald, Score and deviance) are introduced. Effect modifications (a.k.a. interactions) and their meaning in a health context are explored. Model summaries lead to the interpretation of GLMs from the dual perspectives of estimation and Neyman Pearson hypothesis testing (p-values). Finally, ‘standard’ biostatistical techniques (for example t-test, ANOVA, ANCOVA, odds ratios) are revealed to be special (simplified) special cases of the GLM.
The main processes used to drive the content will be a flipped classroom using short instructional videos covering the content, supplemented by extensive online adaptive tutorials, and face-to-face workshops. Active and self-directed learning will be supported via the Moodle TELT.
2. Appraise model fit using a variety of model diagnostics.
3. Compose narratives of GLM interpretation within the framework of statistical inference.
4. Visualise ‘standard’ statistical techniques as special simplified cases of the GLM.
Web-based online learning activities: 7 hours