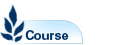
Health Data Analytics: Machine Learning and Data Mining - HDAT9500
Faculty: Faculty of Medicine
School: School of Medical Sciences
Course Outline: MSc Health Data Science
Campus: Sydney
Career: Postgraduate
Units of Credit: 6
EFTSL: 0.12500 (more info)
Indicative Contact Hours per Week: 10
CSS Contribution Charge: 3 (more info)
Tuition Fee: See Tuition Fee Schedule
Further Information: See Class Timetable
View course information for previous years.
Description
Machine learning is the procedure of applying automated algorithms to data, in order to create knowledge. Investigating data in such a manner is often referred to as data mining. In general, the objective of data mining can be classified into one of four categories; pattern analysis, visualising data, pre-processing (e.g. dimensionality reduction), or outlier detection.
Dependent of the specific objective and data, machine learning can be supervised or unsupervised, involving techniques of classification, regression, clustering, and/or association rules. Further, various methods seek to optimise the training error rate.
This course will introduce machine learning through a series of health applications. Each application will pose a set of questions to be addressed. Machine learning techniques will then be applied, generating the knowledge required to address the contextual question. Once the student has mastered the contextual application, the underlying supporting theory will be presented.
2. Design machine learning tasks for Health Data Science scenarios.
3. Construct appropriate training and test sets for health research data.
4. Generate knowledge via the application of machine learning techniques to health data.
5. Appraise methods of training error rate optimisation.
Web-based online learning activities: 7 hours